Keeping up with an industry as rapidly changing as AI is a challenge. So until AI can do it for you, here’s a quick recap of recent stories in the world of machine learning, as well as notable research and experiments that we couldn’t cover on our own.
This week on AI, DeepMind, the Google-owned AI research and development lab. paper We propose a framework for assessing the social and ethical risks of AI systems.
The timing of the paper, which calls for varying levels of involvement of AI developers, app developers, and “broad public stakeholders” in assessing and auditing AI, is no coincidence.
Next week will see the AI Safety Summit, an event hosted by the UK government. The event brings together international governments, leading AI companies, civil society groups, and research experts to focus on how best to manage risks from the latest advances in AI. Includes generative AI (e.g. ChatGPT, stable spread, etc.). So the UK plan Introducing a global advisory group on AI, loosely modeled on the United Nations’ Intergovernmental Panel on Climate Change. The advisory group is made up of a rotating cast of academics who write regular reports on cutting-edge developments in AI and the risks associated with them.
DeepMind has made its vision very clear ahead of on-the-ground policy discussions at the two-day summit. And, to give credit where credit is due, the Institute will continue to explore his approach to investigating AI systems “at the point of human interaction” and how these systems are used and embedded in society. ing.
A graph showing which people are best suited to evaluate which aspects of AI.
But when weighing DeepMind’s offer, it’s worth looking at how the institute’s parent company, Google, scored in a recent survey. study A study published by Stanford researchers ranks 10 major AI models on how openly they operate.
PaLM 2, one of Google’s flagship text analytics AI models, has 100 It is evaluated on a scale and has a score of only 40 points. %.
Now, DeepMind didn’t develop PaLM 2 — at least not directly. However, the institute has not historically been consistently transparent about its models, and the fact that its parent company falls short of key transparency measures is a sign that some top leaders have called for DeepMind to improve. This suggests that there is not much down pressure.
Meanwhile, DeepMind appears to be taking steps to change the perception that in addition to publicly discussing its policies, it remains mum about the architecture and inner workings of its models. The institute, along with OpenAI and Anthropic, pledged several months ago to provide the UK government with “early or priority access” to its AI models to support evaluation and safety research.
The question is, is this just performance? After all, no one would accuse DeepMind of philanthropy. The institute generates hundreds of millions of dollars in revenue each year, primarily by licensing its research internally to his Google team.
Perhaps the institute’s next big ethics test will be its upcoming AI chatbot, Gemini. DeepMind CEO Demis Hassabis has repeatedly promised that he will rival OpenAI’s ChatGPT in its functionality. If DeepMind wants to be taken seriously on the ethical side of AI, it will need to fully and thoroughly detail not only Gemini’s strengths, but also its weaknesses and limitations. We will be closely monitoring how things develop over the coming months.
Here are some other notable AI stories from the past few days.
- Microsoft’s research has revealed that GPT-4 is flawed. A new Microsoft-related scientific paper explores the “reliability” and toxicity of large-scale language models (LLMs), including OpenAI’s GPT-4. The co-authors found that early versions of GPT-4 could spew out harmful and biased text more easily than other LLMs. It’s tough.
- ChatGPT acquires web search and DALL-E 3: Speaking of OpenAI, the company’s officially released Internet browsing functionality to ChatGPT, some 3 weeks after reintroducing the feature It’s in beta after a few months of hiatus. In related news, OpenAI has moved DALL-E 3 into beta, a month after announcing the latest version of its text-to-image generator..
- Challengers to GPT-4V: OpenAI will soon release GPT-4V, a variant of GPT-4 that can understand images as well as text. However, two open source alternatives beat this. LLaVA-1.5 and Fuyu-8B, a model from well-funded startup Adept. Neither has the capabilities of his GPT-4V, but both have close performance. And importantly, they are free to use.
- Can AI play Pokemon?: Software engineer based in Seattle for the past few years. peter whidden is training a reinforcement learning algorithm to navigate the classic first game in the Pokémon series. So far, it has only reached Cerulean City, but Whidden is confident it will continue to improve.
- AI-powered language instructor: Google is focusing on Duolingo with a new Google search feature designed to help people practice and improve their English speaking skills. Coming to Android devices in some countries in the coming days, this new feature provides interactive speaking practice for language learners translating to and from English.
- Amazon deploys more warehouse robots. At this week’s event, Amazon announced Agility announced that it will begin testing its bipedal robot “Digit” in its facility. But reading between the lines, Bryan writes, there is no guarantee that Amazon will actually start implementing digital in its warehouse facilities, which currently utilize more than 750,000 robotic systems.
- Simulator to simulator: In the same week that Nvidia demonstrated the application of LLM to help write reinforcement learning code to guide simple AI-driven robots to better perform tasks, Meta released Habitat 3.0. The latest version of Meta’s dataset for training AI agents in realistic indoor environments. Habitat 3.0 adds the possibility for a human avatar to share her space in VR.
- Chinese tech giant invests in OpenAI rival: Zhipu AI is a China-based startup that is developing AI models that are comparable to those of OpenAI and other companies in the field of generative AI. announced This week, the company announced that it has raised a total of 2.5 billion yuan ($340 million) so far this year. The announcement comes amid heightened geopolitical tensions between the United States and China, with no signs of abating.
- US stops supplying AI chips to China: Regarding geopolitical tensions, the Biden administration announced a number of measures this week to rein in China’s military ambitions, including further restrictions on Nvidia’s AI chip shipments to China. A800 and H800, two AI chips specifically designed by Nvidia to continue shipping to China; will be hit with a new round of new rules.
- AI-powered reprises of pop songs go viral: Amanda highlights an interesting trend. TikTok account Use AI to make characters like Homer Simpson sing rock songs from the 90s and 00s.smells like teen spirit” Although fun and silly on the surface, there is a dark abyss to this whole practice, Amanda writes.
More machine learning
Machine learning models are constantly driving advances in biology. AlphaFold and RoseTTAFold were examples of how a stubborn problem (protein folding) can be effectively trivialized by the right AI model. Now, David Baker (creator of the latter model) and his colleagues have expanded the prediction process to include more than just the structure of the relevant amino acid chains. After all, proteins exist in a soup of other molecules and atoms, and predicting how a protein will interact with airborne compounds and elements in the body depends on the protein’s actual shape and activity. essential for understanding. RoseTTAFold All Atom This is a major step forward for the simulation of biological systems.
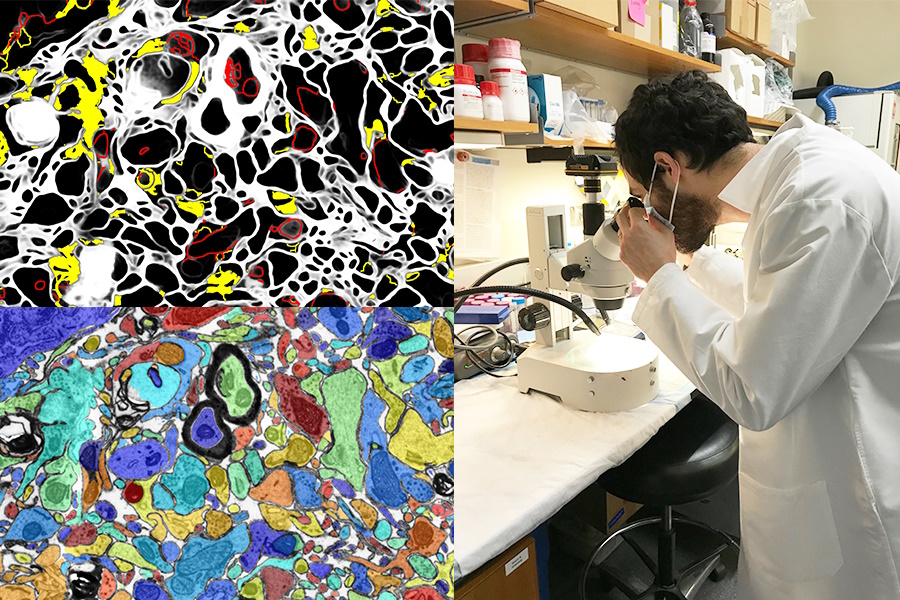
Image credits: MIT/Harvard University
There are also great opportunities for visual AI to enhance laboratory work or serve as a learning tool. SmartEM project by MIT and Harvard University A computer vision system and an ML control system are placed inside a scanning electron microscope and work together to drive the device to intelligently examine specimens. You can also smartly label the resulting images, avoiding less important areas and focusing on interesting or clear areas.
For me, using AI and other high-tech tools for archaeological purposes will never get old (if I may say so). Whether it’s revealing Mayan cities and highways with LIDAR or filling in the gaps in incomplete ancient Greek texts, it’s always cool to see. And this restoration of a scroll thought to have been destroyed in the volcanic eruption that destroyed Pompeii is one of the most impressive ever.
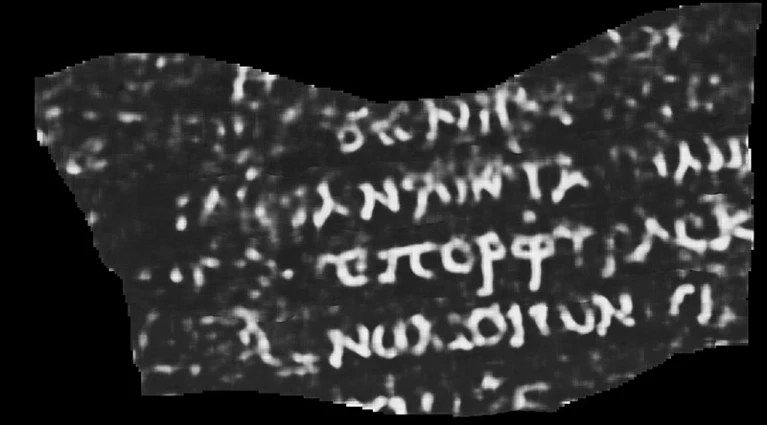
CT scan of burnt and curled papyrus interpreted with ML. The visible letters read “purple”.
Luke Faritor, a CS student at the University of Nebraska-Lincoln, trained a machine learning model to amplify subtle patterns on scans of charred, curled papyrus that are invisible to the naked eye. His method is one of many being tried in an international challenge to read the scrolls, and could be refined to produce valuable academic research. Click here for more information about Nature. What was written on the scroll? Up until this point, only the word “purple” had been used, but that still drove the papyrus scholars insane.
Another academic victory for AI is This system for scrutinizing and suggesting citations on Wikipedia. Of course, AI doesn’t know what is true or factual, but it can gather context about what high-quality Wikipedia articles and citations look like, and scrape the site and web to find alternatives. can. No one is suggesting robots run popular user-driven online encyclopedias, but it could be useful for reinforcing articles that lack citations or that editors aren’t sure about. I don’t know.
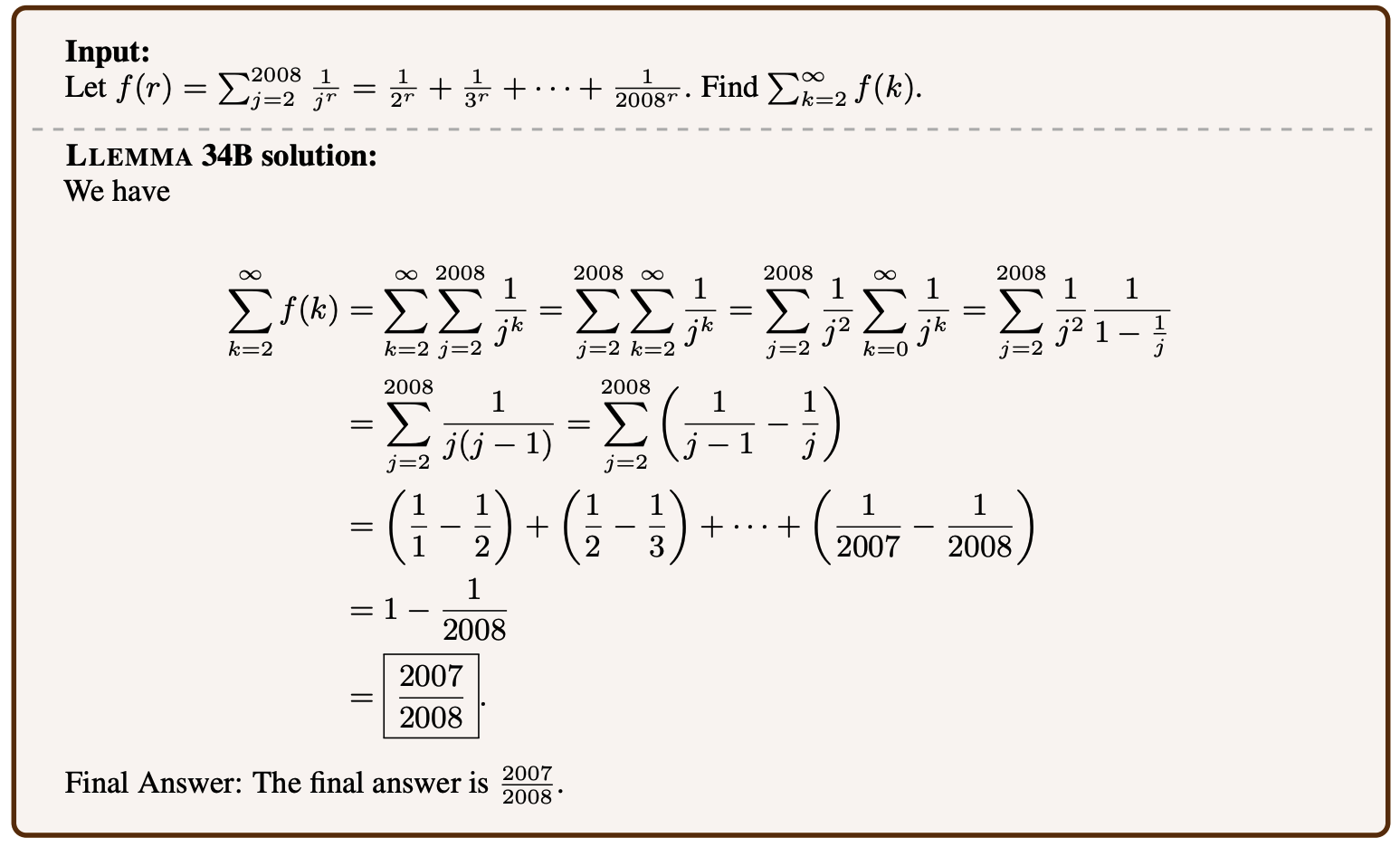
An example of a mathematical problem solved by Llemma.
Language models can be fine-tuned for many topics, and surprisingly, higher mathematics is one of them. Llemma is a new open model They are trained in mathematical proofs and papers that can solve fairly complex problems. This isn’t the first time – Google Research’s Minerva is working on a similar feature – but success and increased efficiency on similar problem sets has shown that “open” models (whatever that word means) It shows that you are competitive. We don’t want a particular type of AI to be dominated by private models, so replicating its capabilities openly is valuable, even if it doesn’t break new ground.
Troublingly, while Mehta has developed his own academic research into mind reading, the way he presents it, like most research in this field, rather exaggerates the process. The paper “Brain Decoding: Toward Real-Time Reconstruction of Visual Perception” states: They may seem to read minds straight.
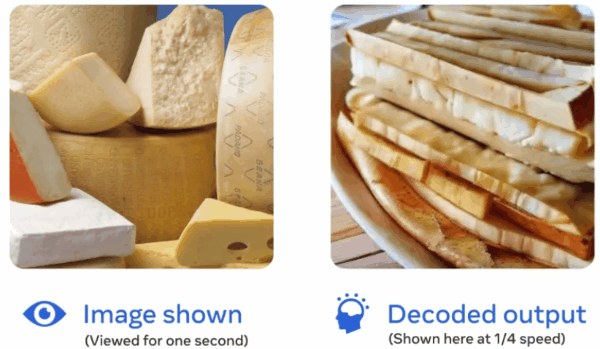
An image that is shown to a person (left) and an image that the AI that generates and infers what the person recognizes (right).
But it’s a little more indirect than that. By studying what high-frequency brain scans look like when people look at images of specific things, such as horses or airplanes, researchers are able to determine what people are thinking and what they’re looking at. Now you can reconfigure it in near real time. . Still, generative AI could play a role here in how we can create a visual representation of something, even if it doesn’t directly correspond to scanning.
should But if it were possible, would we use AI to read people’s minds? Ask DeepMind — see above.
Finally, while the project at LAION is more ambitious than concrete at this point, it is still worthy of praise. Multilingual Contrastive Learning for Speech Representation Acquisition (CLARA) aims to give language models a deeper understanding of the nuances of human speech. Did you know how we can detect sarcasm and sarcasm from non-verbal signals such as tone and pronunciation? Machines are very bad at this, and this is bad news for human-AI interactions. CLARA uses a library of audio and text in multiple languages to identify several emotional states and other nonverbal “speech comprehension” cues.