Researchers from Kyushu University have presented “QDyeFinder,” an AI tool that uses advanced color coding and machine learning to enhance neuron mapping in the brain, demonstrating its potential for wide-ranging applications in biology.
The researchers developed an AI pipeline called “QDyeFinder” that can untangle and reconstruct the brain’s dense neural networks.
The brain is the most complex organ ever created. Its functions are supported by a dense network of tens of billions of neurons, with trillions of connections exchanging information and performing calculations. Trying to comprehend the brain’s complexity can make your head spin. Yet, if we want to understand how the brain works, we need to map its neurons and study how they are wired.
the current, Nature CommunicationsResearchers at Kyushu University have developed a new AI tool, QDyeFinder, that can automatically identify and reconstruct individual neurons from images of mouse brains. In this process, neurons are tagged with an ultra-multicolor labeling protocol, allowing the AI to automatically identify neuronal structures by matching similar color combinations.
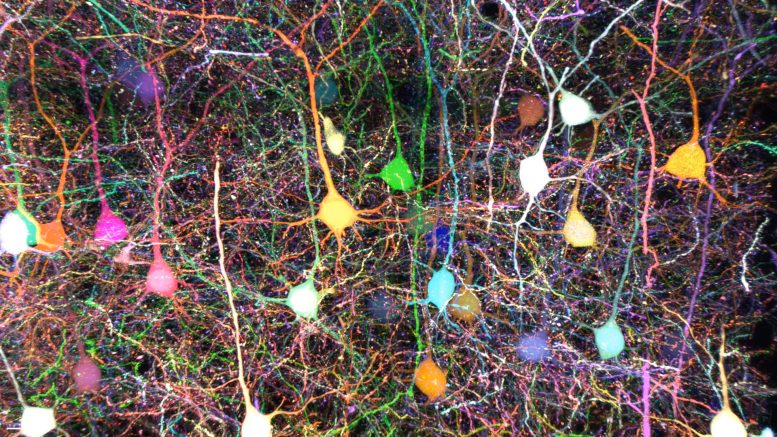
Photo courtesy of Kyushu University/Imai Takeshi
Challenges of Neuronal Mapping
“One of the greatest challenges in neuroscience is mapping the brain and its connections. But neurons are so densely packed together that it is very difficult and time-consuming to distinguish neurons with their axons and dendrites – extensions that send and receive information from other neurons – from one another,” explains Professor Ken Imai of the Graduate School of Medicine, who led the study. “To put it in perspective, axons and dendrites are only about one micrometre thick – 100 times thinner than a standard human hair – and the space between them is even smaller.”
One strategy for identifying neurons is to tag cells with fluorescent proteins of specific colors. Researchers can then track the colors and reconstruct neurons and their axons. By expanding the range of colors, it would be possible to increase the number of neurons that can be tracked at once. In 2018, Imai and his team developed Tetbow, a system that can brightly color neurons with the three primary colors of light.
Mouse cortical layer 2/3 pyramidal neurons were labeled with 7-color Tetbow. A combination of seven fluorescent proteins (mTagBFP2, mTurquoise2, mAmetrine1.1, mNeonGreen, Ypet, mRuby3, tdKatushka2) was used to visualize the dense wiring of neurons. The 7-channel images were then analyzed with the QDyeFinder program to reveal the wiring patterns of individual neurons. Credit: Kyushu University/Takeshi Imai
“The example I often use is the Tokyo subway map. The subway system has 13 lines, 286 stations, and spans more than 300 km. Each line is color-coded on the subway map, making it easy to see which stations are connected,” explains Marcus N. Reiwe, then an assistant professor and one of the paper’s first authors. “Tetebou makes it much easier to trace neurons and discover their connections.”
But two major problems remained: neurons still had to be meticulously traced by hand, and using only three colors was not enough to identify larger populations of neurons.
Innovation with QDyeFinder
The team worked to increase the number of colors from three to seven, but at the time the limitations of human color vision posed a major problem: if you look closely at a television screen, you’ll see that pixels are made up of three colors: blue, green, and red. Because our eyes have blue, green, and red sensors, all the colors we can see are combinations of these three colors.
“Machines, on the other hand, have no such limitations, so we set out to develop a tool that could automatically distinguish between these huge number of color combinations,” continues Leiwe, “and also automatically connect neurons and axons of the same color to reconstruct their structure. We called this system QDyeFinder.”
QDyeFinder first automatically identifies axon and dendrite fragments within a given sample, then identifies color information for each fragment, and then uses a machine learning algorithm developed by the team called dCrawler to group the color information and identify axons and dendrites from the same neuron.
“We compared the QDyeFinder results with data from manually tracked neurons and the results were nearly identical. Accuracy“Compared to existing tracing software, Machine LearningQDyeFinder was able to identify axons with much higher accuracy.”
The team hopes that this new tool will advance the ongoing quest to unravel brain connections, and also hopes to see whether the new technique can be applied to label and track other complex cell types, such as cancer or immune cells.
“Perhaps one day we will be able to read the connections in the brain and understand what they mean or represent for a person. Although I don’t think that will happen in my lifetime, our research represents a concrete step toward understanding the most complex and mysterious aspects of our existence,” Imai concludes.
Reference: “Automatic neuron reconstruction by ultra-multicolor tetbow labeling and threshold-based hue clustering” by Marcus N. Reiwe, Satoshi Fujimoto, Shunichi Baba, Daichi Moriyasu, Viswanath Saha, Richi Sakaguchi, Shigenori Inagaki, and Ken Imai, 25 June 2024, Nature Communications.
DOI: 10.1038/s41467-024-49455-y
Supported by: Japan Agency for Medical Research and Development, Japan Science and Technology Agency, Japan Society for the Promotion of Science, Uehara Memorial Foundation, Sumitomo Foundation, Kanehara Ichiro Foundation, Daiichi Sankyo Life Science Foundation, Brain Science Foundation