We show that an autoregressive language model can learn text embeddings after applying a simple transformation to the dataset. This simply moves a range of text from the middle of the document to the end. Although this data augmentation has received a lot of interest in recent years, there is extensive evidence that training models using large portions of data transformed in this way does not compromise the original left-to-right generation ability. It offers. This is measured by overall disruption and sampling ratings. Wide scale. Considering the usefulness, simplicity, and efficiency of training intermediate imputation (FIM) models, we recommend that future autoregressive language models be trained with FIM by default. To achieve this objective, we perform a series of ablations on key hyperparameters such as data transformation frequency, transformation structure, and filling span selection method. We use these ablations to prescribe strong default settings and best practices for training FIM models. We have released the best filling model trained with API best practices and released a filling benchmark to aid future research.
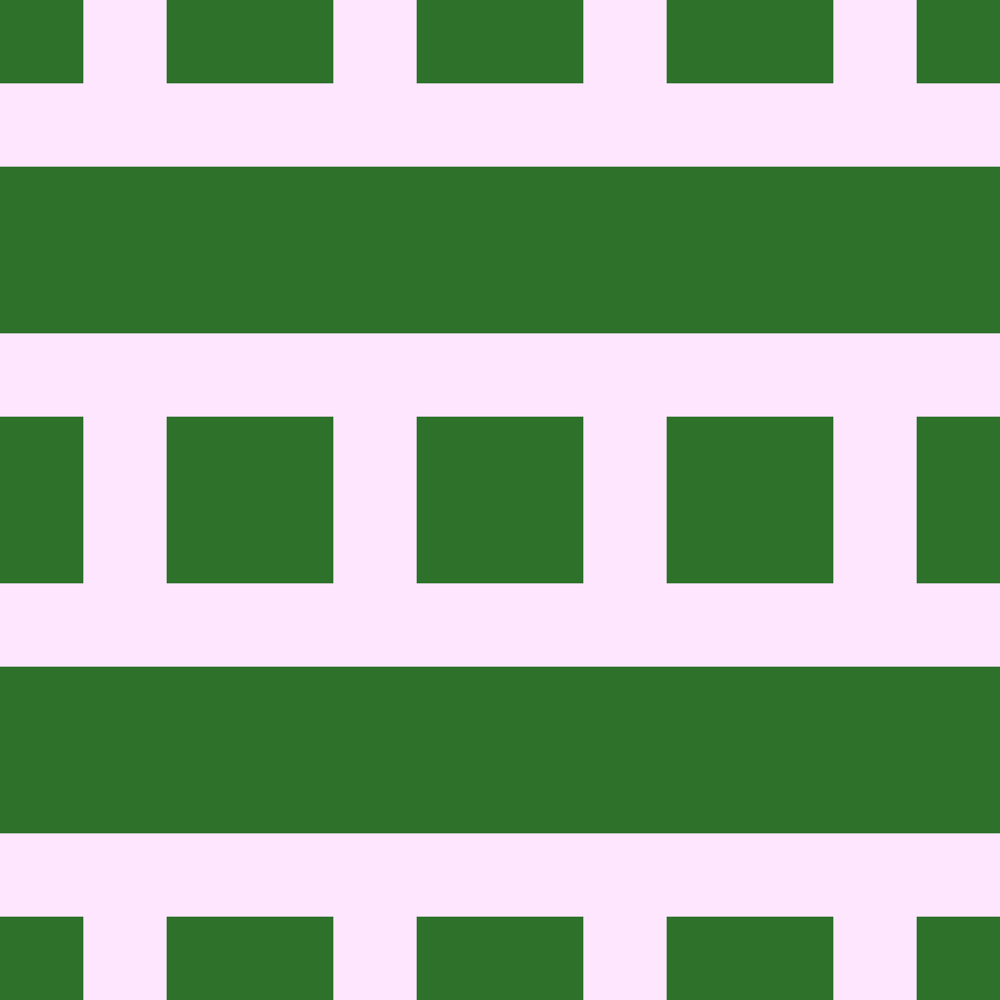