Percentage of planet candidates flagged as contaminants (top) and actual contaminants (bottom) as a function of PC score thresholds for the seven validation sectors. For each validation sector, we chose a threshold that maximized the fraction of contaminants discarded and did not discard any planets. We then performed a weighted average of the thresholds for each validation sector to select the final threshold (dashed line). Weights were assigned as the inverse square of the number of contaminants found in a given sector, allowing for a more conservative cutoff. — astro-ph.EP
Distinguishing between real transit events and false positive signals in photometric time series data is a bottleneck in identifying transiting exoplanets, especially those with long periods.
This distinction typically requires visual inspection of numerous transit-like signals to eliminate instrumental and astrophysical false positives that mimic planetary transit signals. We build a 1D Convolutional Neural Network (CNN) to separate eclipsing binaries and other false positives from potential planet candidates, reducing the number of light curves that require human review.
Our CNN is trained using TESS light curves identified by Planet Hunters citizen scientists as likely to contain transits, including background flux and centroid information. Light curves are visually inspected and labeled by project scientists and undergo minimal pre-processing, only normalization and data augmentation before training.
The median percentage of contaminants flagged across test sectors is 18%, with a maximum of 37% and a minimum of 10%. Our model retains 100% of planets in 16 of the 18 test sectors, but misflags one planet candidate in one sector (0.3%) and two planet candidates in the remaining sectors (0.6%). Our method demonstrates the potential to reduce the number of light curves requiring manual verification by up to a third, while minimizing misclassification of planet candidates.
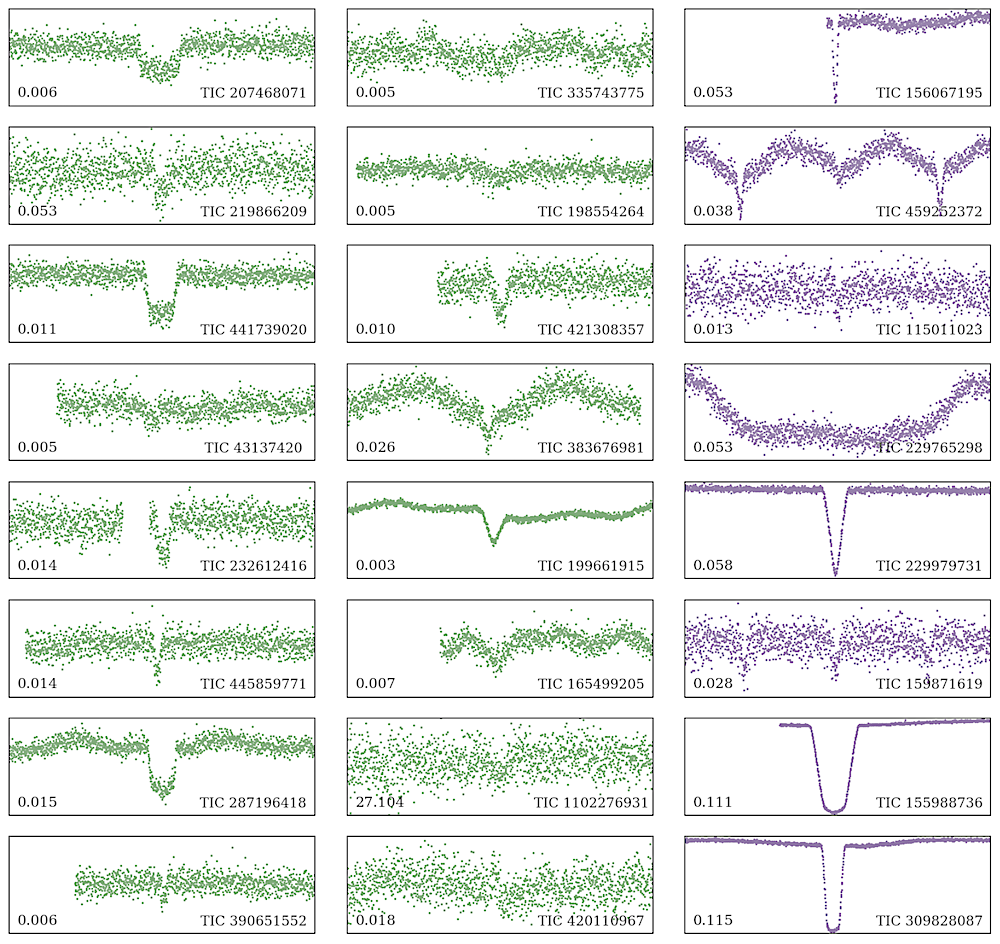
Selected light curve chunks from Sector 50. The right-most column (purple) contains light curves flagged by the model as contaminants. The left-most and middle columns (green) contain light curves classified as “kept for further scrutiny”. The left column shows actual planet candidates, the middle column shows actual contaminants that were incorrectly classified as “kept for further scrutiny”. The y-axis range is shown in the bottom left corner of each panel, and the light curve’s TIC ID is shown in the bottom right corner. — astro-ph.EP
Valentina Tardugno Poleo (NYU), Nora Eisner (CCA), David W. Hogg (NYU, CCA)
Comments: Under review by The Astronomical Journal
Subjects: Earth and Planetary Astrophysics (astro-ph.EP), Astrophysical Measurements and Methods (astro-ph.IM), Machine Learning (cs.LG)
Source: arXiv:2405.18278 [astro-ph.EP] (or arXiv:2405.18278v1 [astro-ph.EP] For this version
https://doi.org/10.48550/arXiv.2405.18278
Focus on learning more
Submission History
By Valentina Tardugnio Poleo
[v1] Tuesday, May 28, 2024 15:29:40 UTC (1,154 KB)
https://arxiv.org/abs/2405.18278
Astrobiology